With AI/ML chip designs containing between 5 billion and 10 billion gates, design verification using hardware emulation is the answer, although not all hardware emulators are the same
Artificial intelligence/machine learning (AI/ML) is the ultimate hot topic of 2019, taking hold of chip design and the semiconductor industry’s imagination and not letting go — for good reason. Close to 1,000 startups in China are in the AI/ML space, along with quite a few in the U.S.
Moore’s Law is being rejuvenated by AI/ML after capacity fears slowed it down. Later this year, estimates put AI/ML design capacity between 5 billion and 10 billion gates — huge capacity for a single design. Designs will go back to what Moore’s Law is supposed to do.
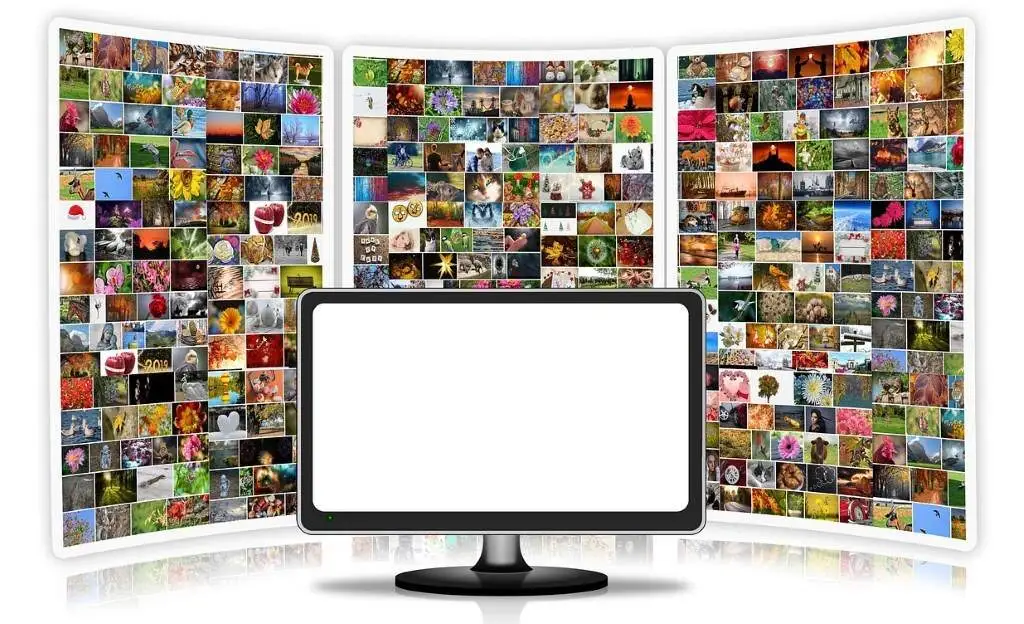
AI/ML is imposing tremendous capacity needs on the market, and these designs also present a challenge to the chip verification market. While the processor may be a relatively simple design, the AI/ML space needs to deploy many of them and scale quickly. Another consideration is software that must be verified along with hardware.
Hardware emulation is the answer, although not all hardware emulators are the same.
One high-profile startup in this market recently announced adoption of hardware emulation for its large AI/ML chip design verification environment. It selected a hardware emulation platform with the largest design capacity commercially available and with a rigorous roadmap for the future. The vendor’s sophisticated and experienced engineers designed the architecture and the chip in-house using their progressive knowledge. The environment has its own operating system and a complete software solution. It offers scalability, capacity, throughput, and a deterministic verification environment. For example, the one-box emulator provided about 2.5 billion gate capacity when it was introduced two years ago. Users today achieve a capacity of 5 billion gates. As capacity needs continue to soar for AI/ML designs and other massively complex chips, the hardware emulation vendor pledges that it will meet the demand with up to 10 billion gate design capacity. In fact, its hardware emulator is deployed now at user sites, enabling determinism to verify large systems.
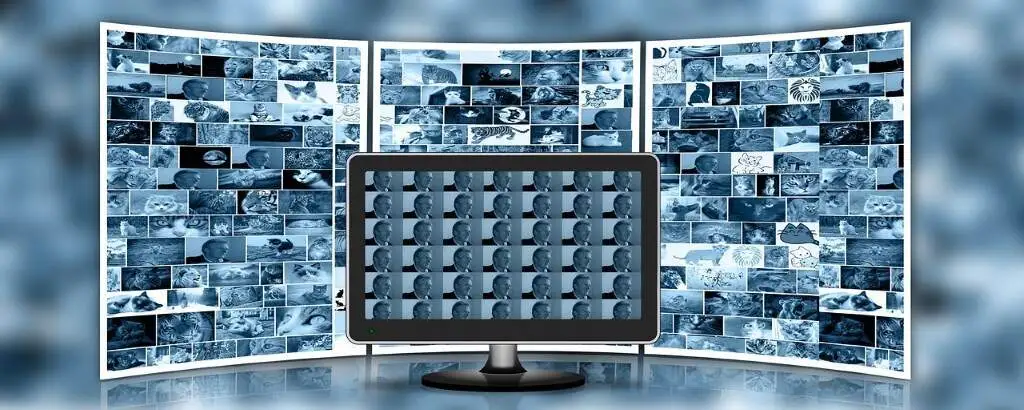
Of course, designers at this startup could have used another emulator if their chip had been smaller and didn’t need to scale or if they didn’t require visibility into the design. As designers start to scale to larger chips, however, they need to run hardware and software and probe with a high amount of visibility. They also need a deterministic environment because an AI/ML design is deterministic, but this is not an option available with all hardware emulation platforms. Another deciding factor is the hardware emulation platform itself, which, in this case, is scalable and supports a virtual system environment because there is no legacy ICE environment that needs to be supported.
Commercially available and competitive hardware emulation platform offerings are different depending on the vendor, so designers need to perform thorough evaluations when it comes to selecting a hardware emulation platform for use in an AI/ML design verification environment. Some approaches may appear to be similar, but they may have fundamental differences “under the hood.” For example, one hardware emulation vendor designs its own chip, but power is a problem as the architecture doesn’t scale well with power as a specification and its roadmap is flawed. Another vendor uses FPGA chips to map the design onto the emulator. This approach has good performance, but design compilation is difficult, as is determinism, and this solution also provides poor visibility into the design.
AI/ML is a vibrant topic in the semiconductor industry, spawning great new product ideas and a great deal of investment. Some hardware emulation vendors are well-positioned to tackle the verification challenge that these new chip designs present, others not so much, so it behooves AI/ML design and verification teams to do their homework before spending a lot of money on a system that doesn’t support the capacities and provide the capabilities demanded by their AI/ML devices.