https://www.gsaglobal.org/forums/the-challenges-to-achieve-level-4-level-5-autonom
Jan Patnzar
Vice President of Sales and Marketing
VSORA
Lauro Rizzatti
Consultant
The path to achieve fully autonomous driving (AD) progresses through five levels of increasing automation, as codified by the Society of Automobile Engineers (SAE) in 2014 under Standard J3016. It starts with L1 or basic driver assistance, and moves to L2, which means feet off the gas or break. L3, as in hands off the wheel, comes next, then L4 or eyes off the road to, finally, L5 that allows all passengers to have their minds off driving. See figure
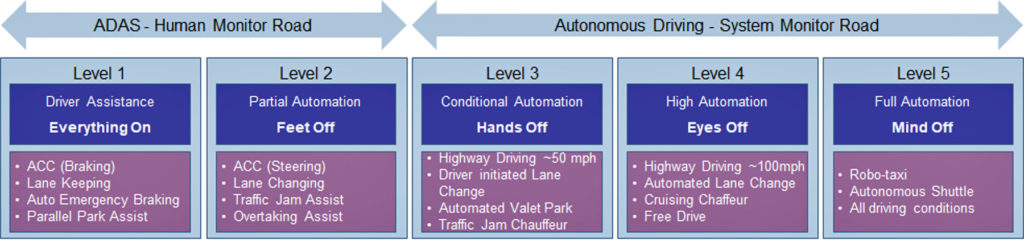
It was anticipated that L3 vehicles would hit the road by the end of the last decade, and early L4 prototypes would be around by 2022. In truth, at the end of 2021, very limited rollouts have been announced so far.
This article illustrates the complexity of the task and examines the requirements necessary to realize L4/L5 vehicles.
The Automated Driving Control Loop
The electronics industry envisioned the implementation of AD vehicles on an architecture consisting of three sub-systems or stages –– perception, motion planning, and motion execution –– called the automated driving control loop. See Figure 2.
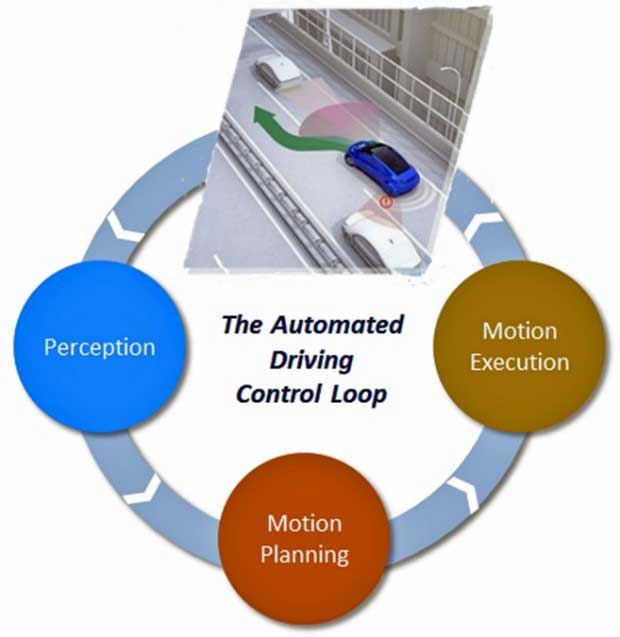
Source: The authors
In the Perception stage, the AD vehicle learns and discerns the surrounding environment by collecting raw data from a comprehensive set of sensors and processing that data via complex algorithms. The data coagulates in the Motion Planning stage into informed decisions that assist the controller to formulate the trajectory of the vehicle. In the Motion Execution stage, the trajectory of the vehicle gets executed autonomously.
Sensors in AD Vehicles
Autonomous driving vehicles require large sets of sensory devices of different types to gather a plethora of information. It’s not only information on static and dynamic objects surrounding the vehicle in motion, including their velocity, i.e., a vector capturing speed and direction. It also detects environmental characteristics, like time of day, weather data, condition of the road, and more.
The collected data, inclusive of historical information, provides the basis for localizing the vehicle, identifying its surroundings, highlighting visible and hidden obstacles and so forth.
As the degree of autonomy increases moving from L1 to L4/L5, the type and number of sensors expand dramatically. They comprise cameras, radars, lidars, sonars, infrared, inertial measurement units (IMUs), and global positioning systems (GPS). It is estimated that in L4 up to 60 sensors will be necessary. See figure 3.
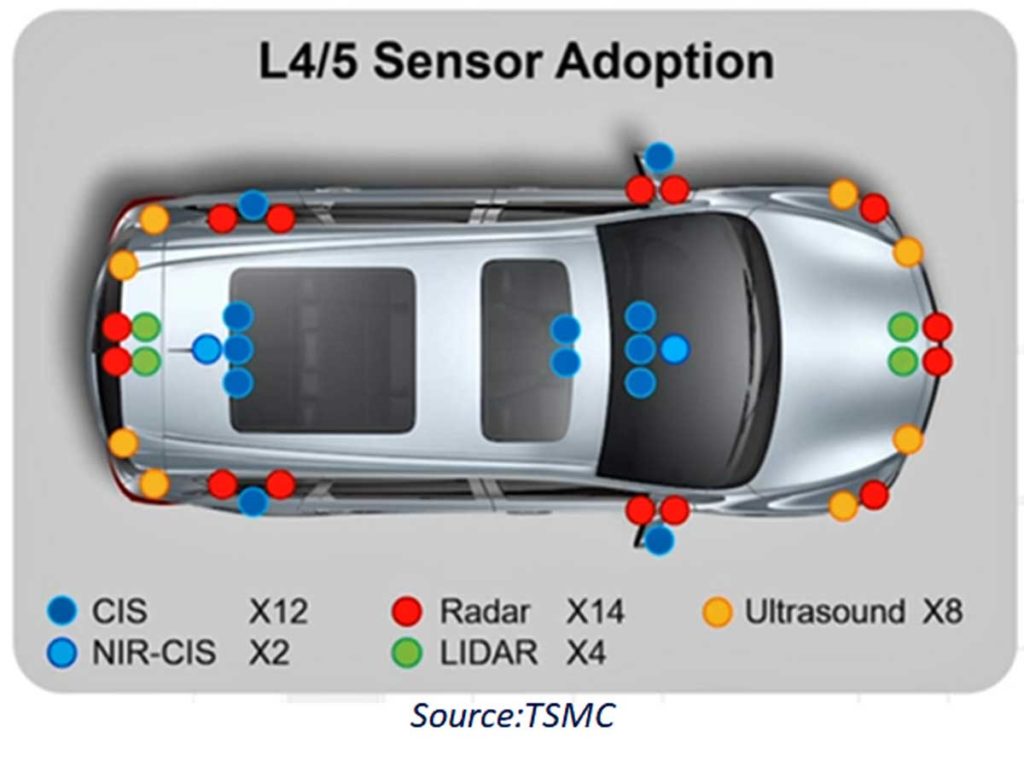
Source: TSMC
Typically, types and numbers of sensors overlap to provide redundant functions for system backup in case of sensor failures. Also, use of advanced data-processing techniques such as sensor fusion process information from multiple sensors simultaneously in real-time improve the perception of the environment.
The sensory data and other data travel within a V2X (vehicle to everything) communication ecosystem via high-bandwidth, low-latency, high-reliability links. This allows cars to communicate to other cars, to the infrastructure, such as traffic lights or parking spaces, to smartphone-toting pedestrians, and to datacenters via cellular networks.
Perception Stage: Key to Achieve L4/L5 AD
Of the three stages, Perception is the critical stage. A successful outcome of control loop operations depends on the ability of the perception stage to understand accurately the environment surrounding the vehicle, including the history preceding the actual reading. Inadequate decoding of the sensory data is a recipe for failure, colorfully captured in the computer vernacular of “garbage-in, garbage-out.”
AD Algorithms
Algorithms processing sensory data are still evolving as new and upgraded algorithms are brought to market on a regular basis pushing the state-of-the-art to new heights.
A key attribute of the perception stage consists of seamless localization with centimeter accuracy. This can be achieved using road environment maps to identify curbs, road markings and structures, for example, assisted by single-scan lidars that generate a vast amount of data, and vision systems where captured data overlays map data.
Emerging algorithms include Enhanced Kalman filters, Unscented Kalman filters and, a glimmering example, Particle filters with the ability to handle both linear and non-liner noise and distribution requiring an order of magnitude larger processing power A Particle Filter, as an example, with a gride size of 280mx280m including eight-million cells of 10cmx10cm sizes and 16-million particles can perform accurate localization of the vehicle and detection of its surroundings, including historical data with a latency of less than 6msec using 1,024 ALUs running at 2GHz.
Requirements to Implement L4/L5 AD Vehicles
Challenges are daunting and need a solution capable of delivering the most formidable set of attributes never encompassed into a single sliver of silicon.
Seven fundamental requirements obstruct the path to success including:
- Massive compute power efficiently delivered
- Very low latency
- Minimal energy consumption
- A combination of artificial intelligence/machine learning (AI/ML) and and digital signal processing (DSP) capabilities
- Deterministic processing
- In-field programmability
- Affordable pricing
All are necessary and none is sufficient. See figure 4.
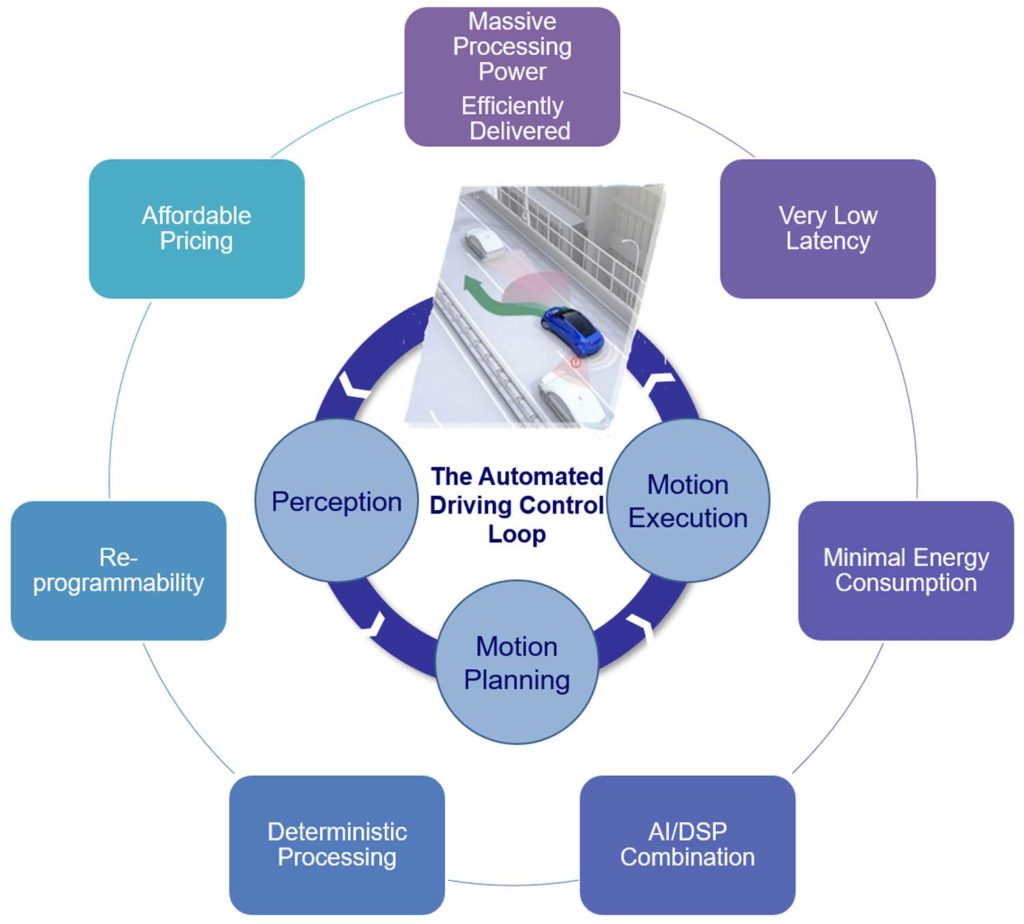
Source: The authors
Massive compute power, efficiently delivered
Moving up the vehicle automation ladder, the processing power requirements increase exponentially from hundreds of GigaFLOPS at L1, necessary to process basic data as in cameras replacing side mirrors, to tens of TeraFLOPS at L2 to implement lane keeping functions. It moves to hundreds of TFLOPS at L3, up to one or more PetaFLOPS at L4/L5.
While all AD processor suppliers specify nominal or theoretical processing power, very few detail the actual or usable power delivered when manipulating sensor data –– that is to say, how much of the available compute power can be used at any given instant. The difference measures the efficiency of an AD processor, expressed in percentage of theoretical power.
What’s needed is efficiency as close to theory as possible, typically 80% or thereabout depending on the specific algorithm.
Very Low Latency
The time elapsed between collecting sensor data and generating informed data must be in the ballpark of 30msec. The threshold leaves about 20msec for the perception stage to accomplish the task.
Longer latency may lead to fatal consequences, as it would occur when a pedestrian crossing a road gets hit by an AD L4 vehicle.
Minimal Energy Consumption
A control loop silicon device installed in an AD vehicle is powered up by the vehicle’s battery. It is critical to design such a device to ensure low average power consumption to avoid draining the battery, as well as low peak power consumption to prevent overheating of the device.
Typically, power consumption ought to be limited to less than 100Watts.
Combination of AI/ML and DSP Capabilities
Currently, AI algorithms in the form of ML and neural network computing dominate the field. While necessary, they are not sufficient.
The adoption of a wide variety of sensor types in large numbers calls for algorithms that combine AI/ML with DSP processors, tightly coupled to limit latency and reduce power consumption.
In the future, more of the same should be expected.
Deterministic Processing
At L4/L5, safety and security concerns will play a fundamental role. AI algorithms deliver approximate responses with less than 100% accuracy, raising doubts and uncertainty and ultimately undermining the approval from the Department of Justice (DOJ).
New algorithms should ensure 100% deterministic responses, complementing AI algorithms with DSP processing.
In-field Programmability
The algorithm technology is a field in evolution. Today’s state-of-the-art algorithms will most likely be modified or not used in two or three years. The ability to handle new algorithms when they become available is crucial for long-term viability, making re-programmability of the silicon in charge of the AD control loop a vital requirement.
More to the point, the move to software-defined vehicles (SDV) will transform the deployment and use of AD vehicles once they leave the factory. The software will be periodically updated over-the-air (OTA), whether to expand the vehicle functionality or to improve its security or add safety-related features.
Affordable Pricing
Commercial vehicles are consumer products in a highly competitive market. Even in the luxury category, AD capabilities must be low cost to ensure the success of a vehicle. Pricing is another critical requirement added to the list of key “must haves.”
A silicon AD processor will be a market success if its price is less than $100 in volume.
Existing AD Silicon Processors
While taken individually, each requirement can be met by existing computing devices. No known device meets all specifications in one sliver of silicon.
Computing devices can be grouped in four categories: CPUs, GPUs, FPGAs, and custom processors.
CPUs do not deliver massive computing power, are inefficient, consume energy, and are costly.
While state-of-the-art GPUs may promise PFLOPS of compute power, their efficiencies range between 10-20% when deployed in AD control loops. Conceived for high parallel processing capacity such as graphic tasks, their efficiency drops to 10-20% delivery only 100-200TFLOPS when elaborating AD algorithms. While latency may be acceptable, power consumption is a drag. Currently, none support DSP capabilities.
While FPGAs deliver middle-of-the-road processing power with low latency and limited power consumption (they run at less than 1GHz), even the latest, best-in-class FPGA generations cannot deliver PetaFLOPs necessary for L4.
The solution to the problem can only come in the form a custom processor designed with an architecture purposely devised for AD applications to meet all seven requirements.
Conclusions
Designing a silicon device for AD control loops mandate a cutting-edge architecture that can meet the level of low latency with PetaFLOPs processing power and 80+% efficiency required for L4/L5 autonomous driving vehicles consuming less than 50 Watts at less than $100.
To add to the challenge, the architecture must combine AI/DSP capabilities sharing data via a tightly coupled on-chip, wide-bandwidth memory, perform deterministic processing, and be OTA re-programmable to accommodate new algorithms.
Only custom processors can meet all seven requirements.
Editor’s note: An example of a custom processor is the VSORA’s Tyr™ based on the VSORA AD1028 architecture, recipient of the “2020 Best Processor IP Analysts’ Choice Award” by The Linley Group.
Jan Pantzar
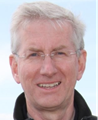
Jan Pantzar is VP Sales and Marketing of VSORA, a provider of high-performance silicon intellectual property (IP) and chip solutions for artificial intelligence, digital communications and advanced driver-assistance systems (ADAS) applications based in France. Mr. Pantzar gained extensive experience in the semiconductor, IP and software industry through building and managing organizations around the globe. Previous experience includes executive positions at Ericsson Corporation (Sweden), Voice Signal Technologies, DiBcom, Cypress and STMicroelectronics He acquired a MSEE degree from Lund Institute of Technology in Sweden.
About Lauro Rizzatti
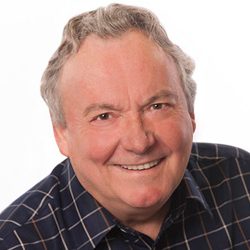
Dr. Lauro Rizzatti is a verification consultant and industry expert on hardware emulation. Previously, Dr. Rizzatti held positions in management, product marketing, technical marketing and engineering.