Despite seven decades of mostly unsuccessful investigation, AI has experienced significant growth over the last 10 years, expanding at an exponential rate. This escalating adoption has been propelled by a shift toward highly parallel computing architectures, a departure from conventional CPU-based systems. Traditional CPUs, with their sequential processing nature that handles one instruction at a time, are increasingly unable to meet the demands of advanced, highly parallel AI algorithms.
A case in point: LLMs. This challenge has driven the widespread development of AI accelerators—specialized hardware engineered to dramatically enhance the performance of AI applications.
AI applications involve complex algorithms that include billions to trillions of parameters and require integer and floating-point multidimensional matrix mathematics at mixed precision ranging from 4-bits to 64-bits. Although the underlying mathematics consists of simple multipliers and adders, they are replicated millions of times in AI applications, posing a sizable challenge for computing engines.
AI accelerators come in various forms including GPUs, FPGAs and custom-designed application specific integrated circuits (ASICs). They offer dramatic performance enhancements over CPUs that result in faster execution times, as well as more efficient model deployment and scalability to handle increasingly complex AI applications.
The AI accelerator market is booming thanks to the widespread adoption of AI across a variety of industries. From facial/image recognition and natural language processing to self-driving vehicles and generative AI (GenAI) elaboration, AI is transforming how we live and work. This revolution has spurred a massive demand for faster, more efficient AI processing, making AI accelerators a crucial component of the AI infrastructure.
Notwithstanding the tremendous market growth, all existing commercial AI processing products have limitations, some more significant than others. AI processing can occur in two primary locations: in the cloud (data centers) or at the edge, each with distinct requirements and challenges.
AI processing in the cloud
The AI accelerator market within data center applications is highly polarized, with one dominant player controlling approximately 95% of the market. To foster greater diversification, a few key issues must be addressed.
- Massive processing power: The processing power must achieve multiple petaFLOPs delivered consistently under real-world workloads.
- High cost of AI hardware: The steep price of AI hardware restricts access for smaller enterprises, limiting adoption to the largest corporations.
- Massive power consumption: AI accelerators consume significant power necessitating expensive installation facilities. These facilities contribute to substantial operational costs, making scalability difficult.
- Market monopoly: By controlling the market, the dominant player stifles competition and prevents innovation. More energy-efficient and cost-effective solutions than existing offerings are needed.
It is worth mentioning that there has been a recent shift in data center focus from training to inference. This shift amplifies the need to reduce the cost-per-query and to lower acquisition and operational expenditure.
All the above improvements would not only make advanced AI capabilities more accessible to everyone, but also promote more sustainable technological growth enabling broader adoption across various industries.
AI processing at the edge
In contrast to the AI processing market in data centers, the AI processing at the edge market is highly fragmented. Numerous commercial products from many startups target niche applications across various industries. From a competitive perspective, this scenario is healthy and encouraging. However, there remains a need for a more comprehensive solution.
Edge AI processing faces a different set of challenges, where low power consumption and cost are key criteria, while compute power is less critical.
Processing efficiency and latency: The Cinderellas of AI attributes
While state-of-the-art AI processors are advertised with impressive processing power, sometimes reaching multiple petaFLOPS, their real-world performance frequently falls short. These specifications typically highlight theoretical maximums and overlook the critical factor of processing efficiency—the percentage of the theoretical power achievable in practical applications. When executing leading-edge LLM models, most AI accelerators experience significant drops in efficiency, often to as low as 1-5%.
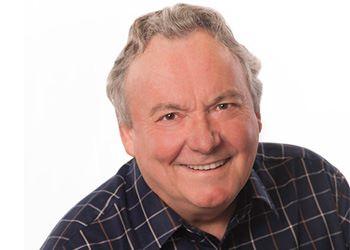
Latency, another crucial metric, is typically missing from AI processor specifications.
This omission arises not only because latency is highly algorithm-dependent but also due to the generally low efficiency of most processors.
Consider two real-world demands:
- Autonomous vehicles: These systems require response times under 20 milliseconds to interpret environmental data collected from a diverse set of sensors. Subsequently, they must decide on a course of action and execute it within 30 milliseconds. These are challenging targets to reach.
- Generative AI: To maintain user engagement, generative AI must produce the first response within a few seconds. To date, this can be achieved by expanding the number of processor accelerators working in parallel. This approach results in significant acquisition costs and operational expenses, with energy consumption becoming a dominant factor.
These scenarios underscore the limitations of commercial processors primarily due to the memory bottleneck that prevents data from being fed to the processing elements fast enough to keep them busy all the time.
A workable solution
To address these challenges and secure a leading position in the market, companies ought to develop next-generation AI accelerators with focus on three primary areas:
- Innovation in technology: A viable solution should be based on a novel AI-specific architecture that defeats the memory bottleneck when the memory cannot deliver data to the multipliers/adders quickly enough. The benefits of higher usable throughput, lower latency, reduced power consumption and cost would be dramatic, leading to leaps in efficiency and broad expansion of their appeal.
- Scalability and flexibility: Developing a scalable, modular and programmable AI accelerator that can handle diverse AI workloads, not just specific tasks, and easily integrate with a variety of platforms and systems could widen the market. This would open the vast area of edge applications from small startups to large enterprises.
- Ease of deployment: A supporting software stack would allow algorithmic developers to seamlessly map their algorithms under development onto the AI accelerator without requiring them to understand the complexities of the hardware accelerator—specifically, RTL design and debugging. This would encourage them to fully embrace the solution.
A winning strategy would also establish strategic alliances with software developers, educational institutions, and other hardware manufacturers to lead to better integration and adoption rates.
—Lauro Rizzatti is a business advisor to VSORA and a noted verification consultant and industry expert on hardware emulation.